Data Science remains an under-determined area of business. Valuable information slips through the fingers of companies, as only a low number of businesses collect and process this data in a conscious manner, e.g. Google, Amazon, Loreal, Bank of America. Which activities in Data Science lie behind the success of these brands? What can you do to enjoy the same benefits from management of your data?
What is Data Science?
Can Data Science be used successfully in business? A large number of companies in the market prove that yes it can. For this reason, in order to understand this phenomenon, we should not only be guided by academic definitions stating that Data Science combines mathematics, statistics, specialised software and advanced analytics with machine learning and artificial intelligence, and that its objective is to investigate a specific issue to be able to identify valuable information hidden in datasets (including business data). Sounds too complicated? No wonder, as the expertise and experiences of the best analytics specialists are behind this statement. You do not need to have professional or specialist knowledge to be able to use Data Science in practice in your business. In this article, and in an easy to understand way, we will show you what Data Science is, and present a few success stories from renown global brands.
First things first. What is Data Science? Just collecting and processing various business data, e.g. information about customers and employees, sales figures, production or stock levels. Most often this information is mixed, contains errors, and is hard to deal with. Now, thanks to advanced algorithms and cloud solutions, you can process, collect and structure even large sets of business data in real time. As a result, you can get the crucial information for taking any business decisions – simple information and conclusions about the real status of an investigated issue.
Data Science algorithms are also practical AI technologies and machine learning methods that use data to not only increase company performance, but also to predict potential future events inside and outside the organisation. This is not reading the tea leaves, but a calculated logical thesis prepared on the basis of historical data and real source data that the company is able to collect.
7 amazing success stories proving that Data Science is essential for your business
Currently, Data Science solutions are so advanced that it is possible to easily develop predictive models, data classification processes and recommendation systems. Business has gained a lot of practical tools that on the basis of data, automatically report and detect anomalies and dependencies that would be difficult for a person to identify. Data Science is already widely used in business. Despite this, a lot of its potential has not been developed yet, and companies are regularly finding new benefits from conclusions provided by good data analyses. A lot of renown brands have noticed the opportunity for development in these, and so use Data Science. Get to know 7 amazing success stories proving that Data Science is essential for your business development too.
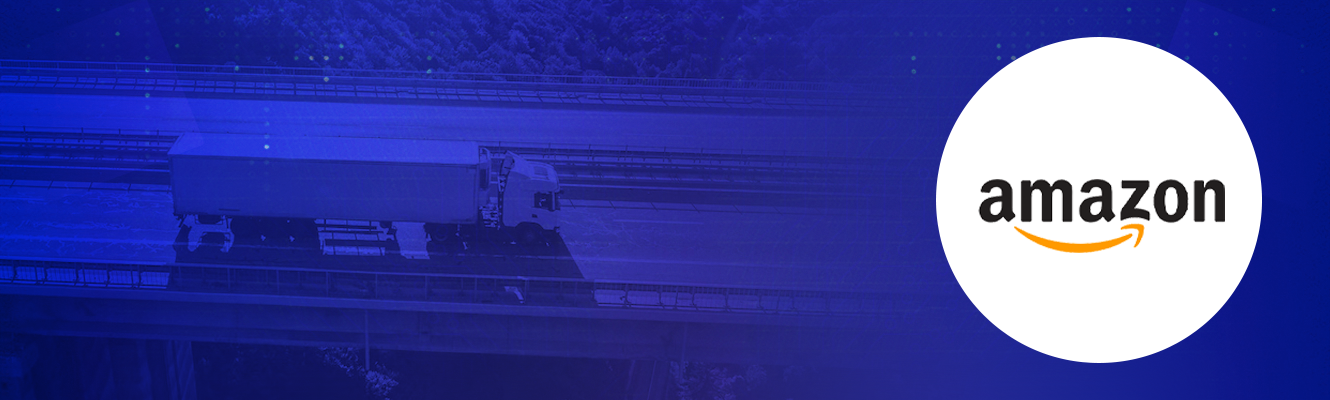
Data Science successes at Amazon
It is safe to say that Amazon shaped Data Science by using the cloud services that we know today from the market. This is also the case with regard to data management in e-commerce. This helps the company to always be in first place in the eyes of customers when they want to find a relevant product. This is not only because Amazon operates on the basis of the “everything in one place” model and has a huge product base, but also due to the fact that all products and recommendations are perfectly adjusted to each customer’s preferences. In this way, this technology generates huge revenues for the company (their share in the market increased to 50% by the end of 2021).
Do you know that Amazon changes prices every 10 minutes on average? The Big Data algorithms of this giant evaluate user readiness to purchase a product on a real-time basis. Prices are established based on user activity on the website, competitor pricing, product availability and more. Data Science enables an immediate response to changes in demand. What is more, almost everything is tracked – from customer needs and their purchase history, to personal details (phone number and address). Addresses help understand the level of user income in order to know which products to offer and which not. Collected data helps in customising shipments. Amazon is famous for rapid deliveries – customers can get their goods on the same day, sent within 5 hours. How does that work? This is all thanks again to data collection. The algorithm predicts what a consumer may order, then the given products are moved to the warehouse closest to the customer’s place of residence.
Amazon uses special software to adjust and analyse customised recommendations for consumers, their Collaborative Filtering Engine (CFE). This algorithm analyses customer purchasing patterns, their likes, their basket, mouseover events, and the time spent on the website. All this information trains the CFE algorithm to better prognose customer purchasing preferences. In practice for example, when you buy bedding, the website can suggest pillows or a blanket in the “Suggested for You” section.
Amazon Kindle is a series of e-readers designed and sold by Amazon. They enable users to browse, buy, download and read electronic books, newspapers, magazines and other digital media, by means of connection to the Kindle Store. The data collection scheme for the Kindle is quite simple. The function of text highlighting and sharing this fragment with others allows analysing user interests and recommending e-books.
Another interesting Amazon function in One-Click Ordering. A lot of people order things online. Searching for products is not as tiring as entering billing and shipping details (especially when you need to enter your credit card details each time). Amazon has resolved this issue with 1-Click Ordering, which allows customers to automatically place orders without taking unnecessary time to again enter personal details. This function is activated when a customer places their first order and fills in their billing and shipping details. After choosing One-Click Ordering, Amazon will find and include their previously entered information. The user still has 30 minutes to change these details. Then, the algorithm checks which products have been ordered, localises them in appropriate warehouses and, if possible, consolidates them in one parcel to avoid paying separate shipping for each product.
Alexa is a voice assistant from Amazon that facilitates everyday life. It is used for simple voice commands. These commands allow finding information e.g. about the weather or the latest news. All the simple voice commands are recorded and then analysed on the Amazon servers in order to tune the algorithm and speech recognition.
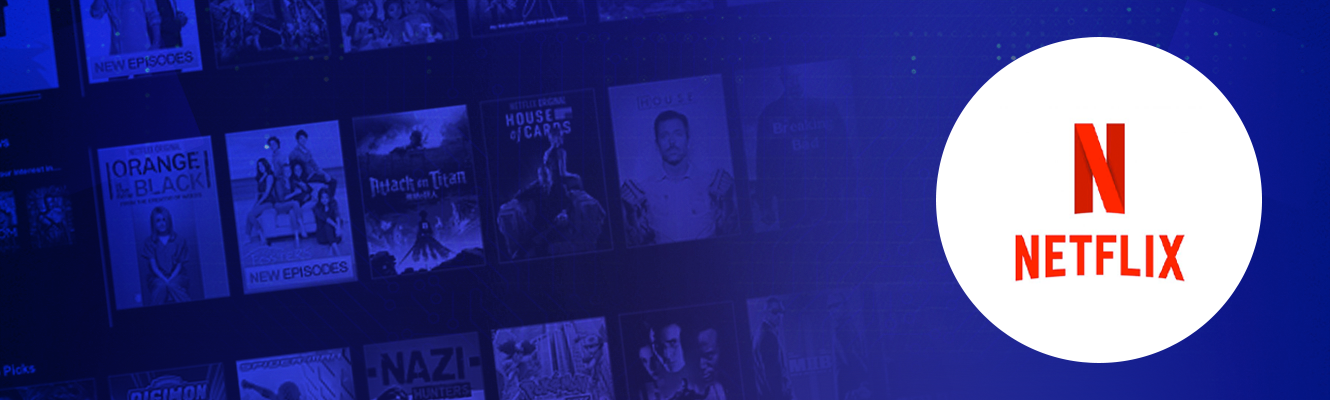
Data Science success at Netflix
Netflix operates on the basis of a recommendation system, i.e. software that delivers content based on user preferences. The algorithm uses this data to predict future user interest. There are two major types of recommendation systems:
Content based filtering – e.g. descriptions, reviews, comments,
Collaborative Filtering – group filtering on the basis of dependencies between user behaviour.
Netflix uses Data Science solutions to process the collected data and conclusions into ranking lists that every user then sees on their profile as suggestions for movies.
Data Science allows Netflix to recommend content and predict popular future content by means of a model that analyses how similar programmes gained popularity in the past.Another Data Science tool that Netflix benefits from is Artwork Visual Analysis (AVA), a set of tools and algorithms designed to choose high-quality imagery from movies. One season of a 10-episode series is about 9 million film frames. People would find it hard to choose the most attractive moments of each series. For this reason, the AVA algorithm was developed, which chooses high-quality shots that are most likely to attract the viewers’ attention.
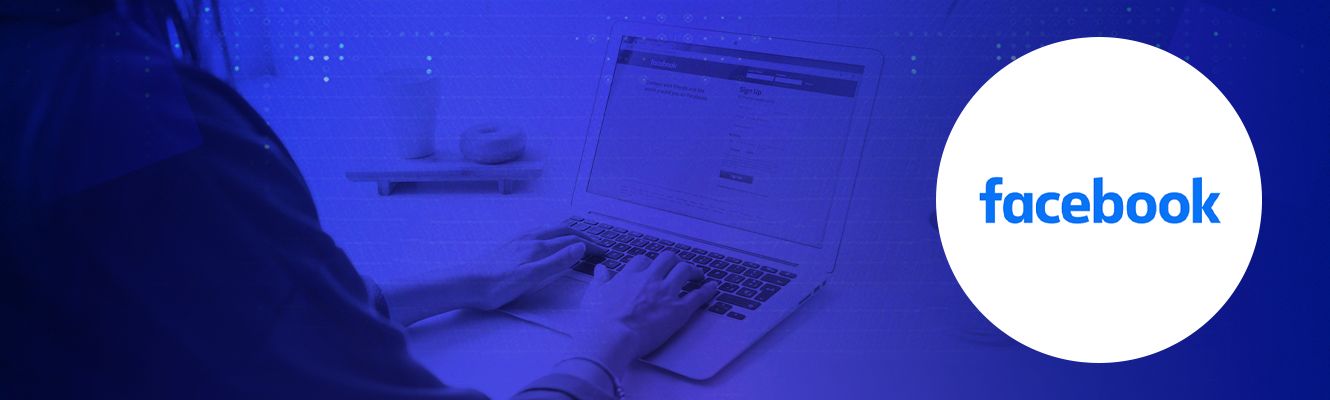
How does Facebook process data from posts?
The majority of data published on Facebook is text data. Meta (former Facebook Inc.) is aware of this and therefore uses an internally developed tool called Deep Text, which analyses and draws conclusions from datasets in the form of texts that we share and publish on this social medium.
Traditional analytics methods require time-consuming pre-treatment based on complex engineer and language expertise. Slang, common expressions and different spellings are additional challenges. Advanced DeepText algorithms and machine learning techniques allow the system to draw conclusions from texts without additional knowledge of the language. The most relevant comments are displayed by DeepText.
Thanks to considerable investments in DeepFace (face recognition technology), Meta can track their users on the entire platform and the Internet. By publishing photos on their profiles or tagging other users, users avail Meta of huge datasets.
As far as ads are concerned, Meta uses Data Science to estimate the ad creations that users will like most. Algorithms for graphic content recognition check the elements a graphic contains, how much of the graphic is taken up by the text itself, and whether promoted content does not breach the platform terms and conditions. Through a comprehensive data analysis of a campaign, after several dozen clicks the system is able to indicate the creation most liked by users and direct it to those people whose personal profiles are the most similar to those who have already clicked on it. It is no secret that the terms we type in the search engine can impact ads that are displayed later. For blind people, Automatic Alternative Text generates automatic descriptions of the graphics. In this way, blind users can browse their information channel and listen to the descriptions of each image stored in the “alt-text” attribute. Alternative text is built on the basis of the “this image can contain…” principle, followed by a list of objects recognised by the system. Data Science helps resolve real issues of the platform users.
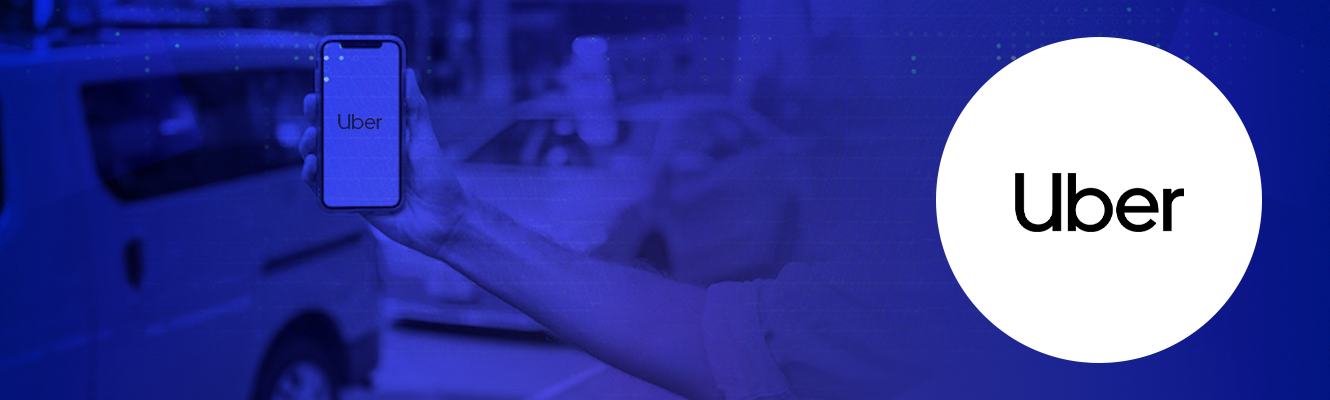
How does Uber use Data Science?
The model of multiplying Uber fare rewards is based on geographic localisation and the demand for services, thanks to which the system can efficiently position drivers. Using a regression analysis, Uber finds out which areas are the busiest at particular times to be able to raise the fare reward in order to draw more drivers.
Uber algorithms track driving conditions and distances in real time, combining them with GPS data to make relevant corrections. Uber fares are based, among others, on driving length. Fare calculation algorithms can be easily adjusted to the transport needs in a given localisation.
Similar models are used by hotel networks and airlines for shaping products and services. The difference is that the Uber predictive modelling serves for estimating demand in real time.
Conscious data management is predicting and preventing anomalies, errors and frauds caused by external factors. For such cases, Uber developed RADAR – a system for detecting and relieving frauds connected with providing or using their services in the application. On the basis of source data collected, the AI system can identify frauds in the initial phase and then generate rules in order to stop or prevent them in the future. The system is able to automatically identify an account that fits the rule assigned e.g. to a model of collecting fraudulent fees for cleaning. After confirming that the user has committed unfair practices, their account is deleted.
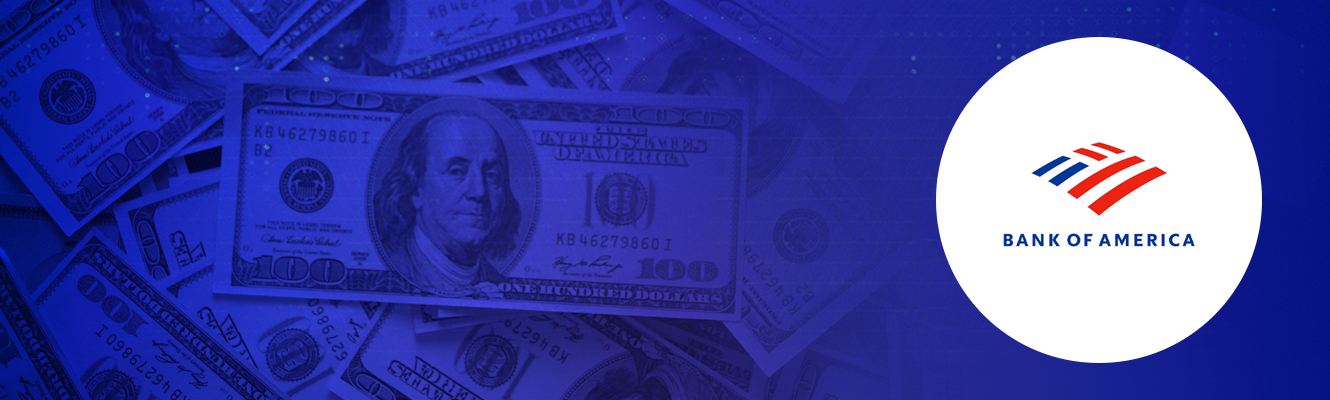
Bank of America and the data
All entrepreneurs know that retaining customers is much cheaper than winning new ones. This is of particular importance in the financial sector, where the number of competitive solutions for traditional banking increases every year. Banks annually invest large sums to make customer service a motivating factor for becoming a regular customer. Bank of America is an excellent example. In June 2018, it presented a virtual assistant Erica based on artificial intelligence. Erica collects and processes data, and then interprets speech to help customers in the quickest way possible. In addition, Erica:
- Warns customers that their behaviours related to expenses are most likely to lead to a nil balance within one week,
- Reminds customers about payments (including overdue payments),
- Advises how to reduce monthly expenses.
In the first quarter of 2021, Erica was used by 19.5 million BofA customers, which indicates that this solution enjoys great popularity. Satisfied customers who consciously manage their finance mean profits for the bank, as well as relative stability of the managed assets.
Scientists dealing with data and engineers from Bank of America developed the Predictive Intelligence Analytics Machine (PRIAM), in which artificial intelligence uses a network of controlled algorithms to understand and predict trends between ECM transactions and investors. PRIAM collects data from the details of actions, histories of previous transactions, market data, and information from points of sale. It is able to collect the data from 1,000 investors within a few seconds.Risk modelling is a set of techniques that indicate the level of risk associated with particular bank transactions and operations in the financial market. The case of credit risk quantification is particularly important, namely the consequences of debtor bankruptcy or market risk. Banks employ experts in risk modelling in order to develop models for reducing the risk of financial losses by the bank. A lot of financial institutions are already using digital solutions for risk modelling. When a person wants to take out a loan, advanced software collects essential data, investigates their loan history and gives a creditworthiness and non-payment risk assessment.
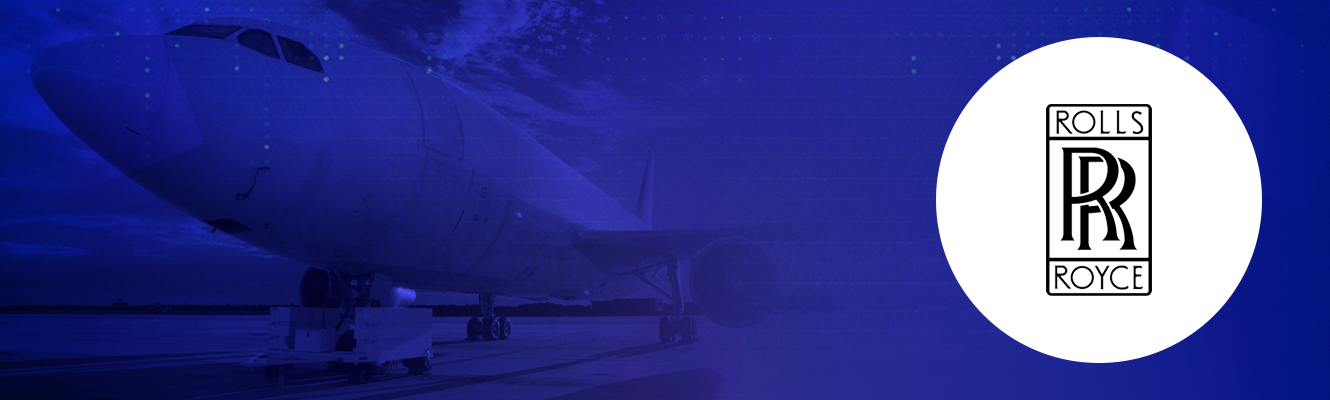
Rolls-Royce good exemple
Data Science is also used in the aviation industry. Systems for booking tickets, registration of luggage and searching for optimal connections are the most obvious uses of collecting and processing masses of data. A less obvious use of Data Science are aeroplane engines. One such project is artificial intelligence that collects data on engines in real time and processes it in the cloud, which considerably reduces the unnecessary maintenance and downtime of aeroplanes. Rolls-Royce was able to shift to a model that allows seeing each part of the engine separately, and developed a mobile application to see in real time when a given part of the engine will need to be exchanged or repaired. All this save nerves, money and even human life.
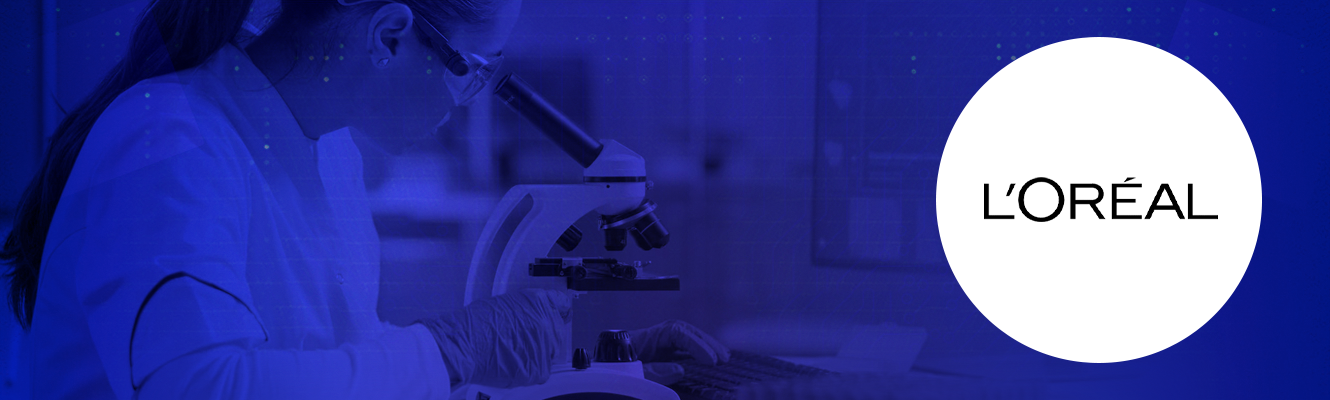
L’Oréal
The globalised cosmetic industry requires a faster implementation of innovations in order to meet the growing desires and needs of customers looking for new products and services. Data Science and artificial intelligence allow faster development of new cosmetic products to satisfy the never-ending stream of variety in customer needs worldwide. A good example is where L’Oréal uses such tools for processing data in the cloud in such areas of research as toxicology, and tracking microorganisms and their influence on the skin.
Does Data Science directly impact business metrics?
A lot of Data Science implementations are not visible at first glance, which is one of the main reasons why many companies ignore this powerful tool. The successes of all of these above-mentioned companies show that collecting and processing data can enable a thorough analysis of data arriving into your company, and they are a practical tool for generating profit.
It is enough to look at the Netflix series recommendation technique, the speech recognition by Facebook, and the product recommendation by Amazon. All of these have been big success stories and have had a massive financial boost. Do you need more? Check out our brief descriptions of the best examples of Data Science that are making money:
LinkedIn feeds mix the topics you have selected and feel you might like, based on what you read and engage with. It’s very predictive and works on cutting-edge machine learning and text analysis solutions. They show you ads related to your interest, job or the industry you work in.
The “Suggested for you” or “Others also Bought” sections in e-stores show perfectly how Data Science helps increase sales. Algorithms use up-selling and cross-selling strategies and discreetly suggest which belt can match the trousers you have just added to the basket.
Thanks to the artificial structure of a neural network, Deep Learning works perfectly in finding patterns in unstructured data, e.g. images, sound, video, text. This Data Science solution is used in a variety of business areas. Systems using artificial intelligence contribute to performing tasks in a more effective and accurate manner than in the case of people. Similar technologies are used for detecting frauds, customer database management systems, recognising faces and elements in graphics in ad management systems, etc.
The advanced technologies of voice assistants are an effective way of collecting data that can later assist in service quality improvements, targeting ads adjusted to the user, and customising presented content. Siri, Google Now and Alexa are indispensable elements of phone software that facilitate life, and at the same time collect data from user profiles and generate huge income for their owners.
How to implement a data science strategy in my company?
Where does the secret of all the presented companies lie? In Data Science.
Each of these stories are proven procedures and tools for collecting, processing, analysing and presenting data in a way that supports business development. At some point, Google, Amazon and Netflix understood the meaning of the incoming data. Maybe it’s your turn?
Benefit from Stepwise experience and expertise. Cutting-edge technologies, effective software, versatile competencies of specialists and good practices directly from the largest global companies allow us to develop and implement a Data Science strategy in a considerably faster manner.
If your company already has data, you need to find out how you can start earning from it. Our data scientists will prepare a data analysis strategy for you, suggest tools that will automate data processing, and deliver specific conclusions or prognoses that will accelerate the development of your business.If you are looking for help in identifying data sources in your company, or wish to find out how you can collect data from users, customers or employees (in accordance with the law), you need a reliable and experienced advisor who can teach you everything. Contact us – we are always easy to talk to! Find out more about our Data Science service.